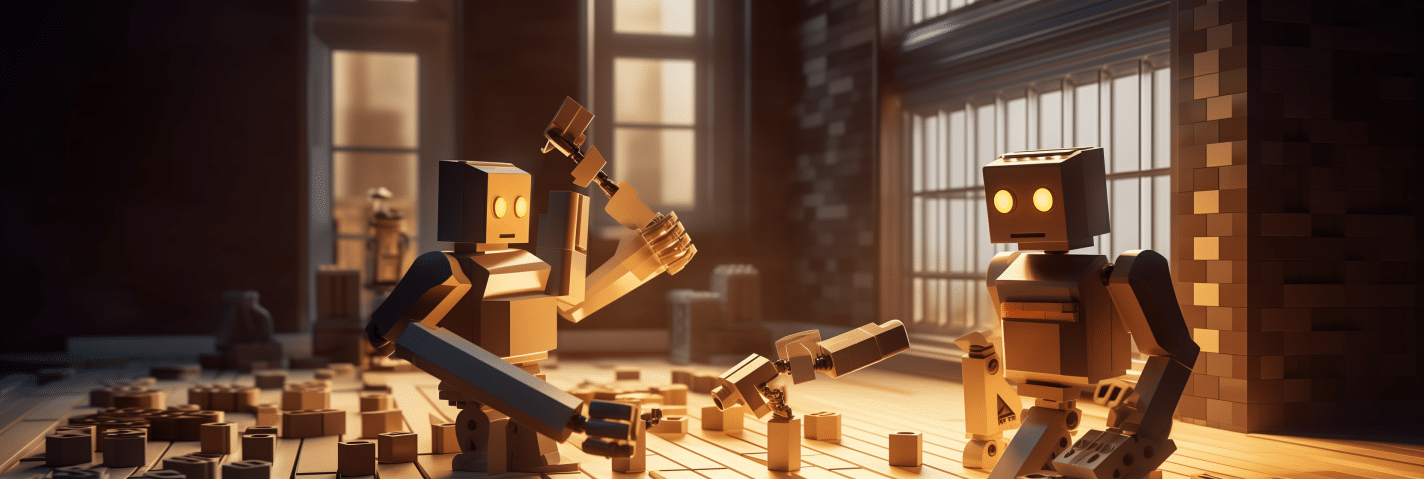
Generative AI for Data Synthetics – Will it Change Testing
January, 2024
by Jane Temov.
Jane Temov is an IT Environments Evangelist at Enov8, specializing in IT and Test Environment Management, Test Data Management, Data Security, Disaster Recovery, Release Management, Service Resilience, Configuration Management, DevOps, and Infrastructure/Cloud Migration. Jane is passionate about helping organizations optimize their IT environments for maximum efficiency.
In the intricate world of software testing, Test Data Management (TDM) stands as a cornerstone for project success. Picture this scenario: you’re gearing up to test a critical software application, and what you need is data that faithfully replicates real-world conditions. Yet, the acquisition and management of such data often prove to be laborious, error-prone processes laden with challenges.
TDM, at its core, is the art of acquiring and managing data for software testing, all while minimizing human intervention. The quality and availability of data are paramount to the triumph of any testing endeavor. Without these, defects might evade detection, and software may fall short of user expectations.
Innovate with Enov8
A Platform of Insight
Managing your IT & Test Environments, Releases & Data.
In this article, we’ll embark on a journey into the realm of TDM, explore its traditional methodologies, and then set our sights on the disruptive landscape of Test Data Synthetics (or Data Fabrication).
Traditional Scope of TDM and Introduction to Data Synthetics
Conventional TDM methods often involve approaches like production database cloning, where data is copied directly from production environments for testing purposes. While this might appear straightforward, it comes with its share of challenges. Foremost among them is the need for data masking to safeguard sensitive information and ensure compliance with data privacy regulations. Furthermore, the costs associated with maintaining duplicate environments can reach astronomical heights.
Enter Test Data Synthetics, a contemporary alternative. Test Data Synthetics, also known as Data Fabrication, is revolutionizing the TDM landscape. Instead of relying on costly and time-consuming cloning processes, Test Data Synthetics harnesses artificial intelligence and generative algorithms to craft synthetic data mirroring the characteristics of real data. This synthetic data isn’t just a game-changer; it’s a savior.
Benefits and Challenges of Test Data Synthetics
The advantages of Test Data Synthetics are manifold. It eliminates the need for data masking and subsetting, as synthetic data, by its very nature, lacks sensitive or confidential information. This significantly streamlines the testing process, reducing both time and costs.
Moreover, Test Data Synthetics offers unparalleled flexibility. It enables the generation of various types of data, from straightforward test cases to intricate edge scenarios, catering to a wide spectrum of testing requirements. Whether you seek to simulate peak usage conditions or obscure corner cases, Test Data Synthetics rises to the occasion.
However, like any innovation, Test Data Synthetics is not without its challenges. A primary concern revolves around ensuring that synthetic data adequately represents real-world scenarios. Inadequate representation can lead to false positives or negatives during testing, ultimately affecting the quality of the software under development. Striking the right balance between data synthesis and realism is an ongoing challenge that organizations must grapple with when adopting this approach.
Application of Test Data Synthetics in Software Development Lifecycle
Test Data Synthetics shines most brilliantly in the early stages of the software development lifecycle. Imagine the agility and flexibility it offers during development and testing. In these phases, rapid and iterative testing reigns supreme, and the capacity to generate diverse, dynamic datasets on-demand becomes a game-changer.
Picture a scenario where your development team needs to simulate user interactions across various environments, from diverse devices to global locations. With Test Data Synthetics, you can effortlessly conjure up the necessary datasets, empowering developers to test and optimize their code in myriad conditions.
Nevertheless, as we advance deeper into the software development lifecycle, especially during integration and user acceptance testing, the need for more accurate and realistic data intensifies. This is where the challenges of employing synthetic data come to the fore. While synthetic data can emulate real data to a significant extent, it may not fully replicate the intricacies of actual user behavior, potentially leading to testing blind spots.
DATACRAFT – AI Based Data Synthetics (part of Enov8 TDM’s DaC Suite)
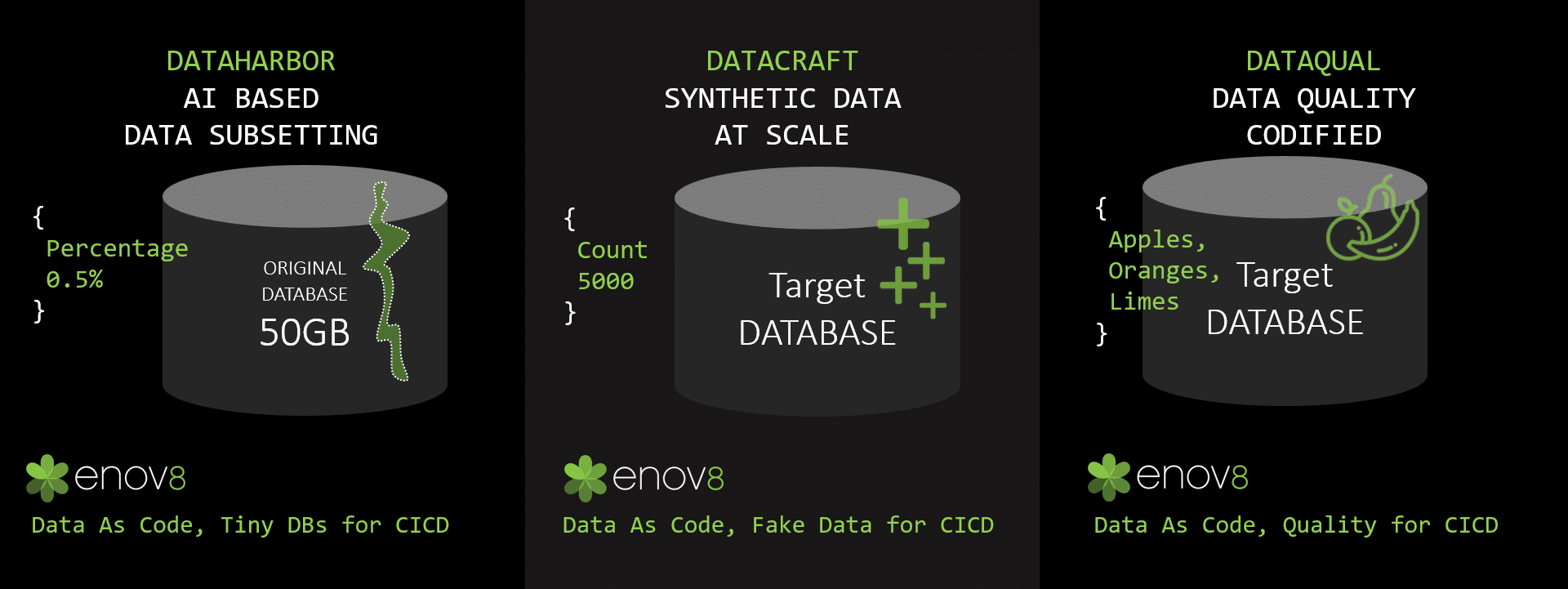
Generative AI in Data Synthetics
The advent of Generative AI serves as the catalyst propelling Test Data Synthetics into new frontiers of sophistication and accuracy. Generative AI algorithms, such as Generative Adversarial Networks (GANs), exhibit the capacity to create data remarkably close to real-world data, in terms of both structure and distribution.
Generative AI takes data generation to a higher echelon. These algorithms analyze existing data, discern its patterns, and subsequently generate synthetic data points that seamlessly blend with genuine data. Such fidelity heralds a significant advantage for software testing.
For instance, contemplate training a machine learning model for fraud detection. With generative AI, you can amass extensive datasets of legitimate and fraudulent transactions, fine-tuning your model to detect even the subtlest anomalies. Such precision and control were previously beyond the grasp of traditional data generation methods.
The potential transformations this brings to software testing and Test Data Management are profound. It not only expedites the testing process but also elevates its effectiveness. In an era where software complexity continues to mount, the ability to create precise testing scenarios represents a monumental leap forward.
Balancing Data Generation with Broader TDM Aspects
While Test Data Synthetics and data generation constitute pivotal components of Test Data Management, they form just one facet of the entire picture. It’s imperative to recognize that TDM encompasses a broader spectrum of responsibilities, all contributing to the quality and reliability of software testing.
Data governance, data compliance, and data provisioning stand as equally vital aspects of TDM. Ensuring ethical data usage, compliance with regulations like GDPR, and the timely provision of data when and where needed pose challenges that organizations must address alongside data generation.
One noteworthy solution in this context is the Enov8 Test Data Manager (Data Compliance Suite), which embraces a holistic approach to TDM. It harmoniously combines data generation with comprehensive data governance and compliance features, ensuring that your testing practices not only yield accurate data but also adhere to legal and ethical standards.
The Role of Companies like Enov8 in Advancing Generative AI
In this exploration of Generative AI’s remarkable potential in Test Data Management (TDM) and software testing, it’s imperative to acknowledge the pivotal role played by forward-thinking companies like Enov8. Enov8, a leader in TDM Solutions, stands at the forefront of integrating Generative AI into their platforms, catalyzing the continuous evolution of TDM practices.
Enov8’s commitment to advancing the industry through cutting-edge technologies is a testament to their dedication to delivering superior solutions to their customers. Their comprehensive approach, exemplified by the Enov8 Test Data Manager (Data Compliance Suite), underscores the importance of striking a balance between data generation and broader TDM aspects.
These companies bridge the gap between innovation and practical implementation, empowering organizations to harness Generative AI’s potential without compromising data quality, security, or ethical considerations. Enov8’s platforms provide a seamless ecosystem where data generation, management, and compliance coexist harmoniously, fostering efficiency, accuracy, and trust in the testing process.
Conclusion
In this comprehensive exploration of Generative AI for Data Synthetics and its potential impact on Test Data Management (TDM) and software testing, we have unveiled a transformative shift in how organizations approach testing and data provisioning.
Test Data Synthetics, fueled by the power of Generative AI, is reshaping the boundaries of TDM. It offers a revolutionary alternative to traditional methods, liberating organizations from the complexities of data cloning, masking, and high infrastructure costs. With Test Data Synthetics, the benefits are clear – faster testing, reduced costs, and unparalleled flexibility in generating diverse data scenarios.
However, it’s crucial to acknowledge that Test Data Synthetics isn’t a one-size-fits-all solution. While it excels in the earlier stages of the software development lifecycle, challenges emerge when precise data representation is essential in later testing phases.
Moreover, Test Data Management extends beyond data generation, encompassing data governance, compliance, and provisioning. Companies like Enov8 lead the way in integrating Generative AI into their platforms, contributing significantly to the continuous evolution of TDM solutions.
In closing, Generative AI and Test Data Synthetics are poised to reshape the future of software testing and Test Data Management. By striking the right balance between innovation and responsibility, organizations can harness these advancements to enhance the efficiency of their testing processes while ensuring ethical and compliant data use in an ever-evolving technological landscape. Embracing these transformative technologies is key to staying competitive and delivering high-quality software products to users worldwide.
Relevant Articles
DORA Compliance – Why Data Resilience is the New Digital Battlefield
How Enov8 Helps Financial Institutions Align with the EU's Digital Operational Resilience Act Executive Introduction As of January 2025, the EU's Digital Operational Resilience Act (DORA) has become legally binding for financial institutions operating across the...
Data Fabric vs Data Mesh: Understanding the Differences
When evaluating modern data architecture strategies, two terms often come up: data fabric and data mesh. Both promise to help enterprises manage complex data environments more effectively, but they approach the problem in fundamentally different ways. So what’s...
What Is Release Management in ITIL? Guide and Best Practices
Managing enterprise software production at scale is no easy task. This is especially true in today’s complex and distributed environment where teams are spread out across multiple geographical areas. To maintain control over so many moving parts, IT leaders need to...
Test Environment: What It Is and Why You Need It
Software development is a complex process that requires meticulous attention to detail to ensure that the final product is reliable and of high quality. One of the most critical aspects of this process is testing, and having a dedicated test environment is essential...
PreProd Environment Done Right: The Definitive Guide
Before you deploy your code to production, it has to undergo several steps. We often refer to these steps as preproduction. Although you might expect these additional steps to slow down your development process, they help speed up the time to production. When you set...
What is Data Tokenization? Important Concepts Explained
In today’s digital age, data security and privacy are crucial concerns for individuals and organizations alike. With the ever-increasing amount of sensitive information being collected and stored, it’s more important than ever to protect this data from...